In the modern retail ecosystem where the consumer is in control of the marketing channels, cloud based contact center can offer your retail business a solution to connect to the consumers across multiple touch points. Cloud based service offerings can not only enhance omnichannel experience, but also streamline data flow and optimize your computing operations.
Recommendation system with purchase data is at the heart of the technological change in the retail sector that has transformed the way retailers interact with their customers. Retailers are now moving away from traditional marketing and finding newer ways to engage with their customers more efficiently and meaningfully by going the digital transformation way.
Recommendation engines encourage customers to explore newer products. They provide better and richer alternatives for items the customers plan to purchase. They also recommend options under various categories like related items, popular products, items bought together, and more.
These experiences are important to keep customers loyal to a retailer and consequently improve the retailer’s ROI. Netflix may not be a traditional retailer, but it is easy to understand how lost the viewers would be had it not been for its super-efficient, ever evolving recommendation system!
Recommendation Systems with Purchase Data
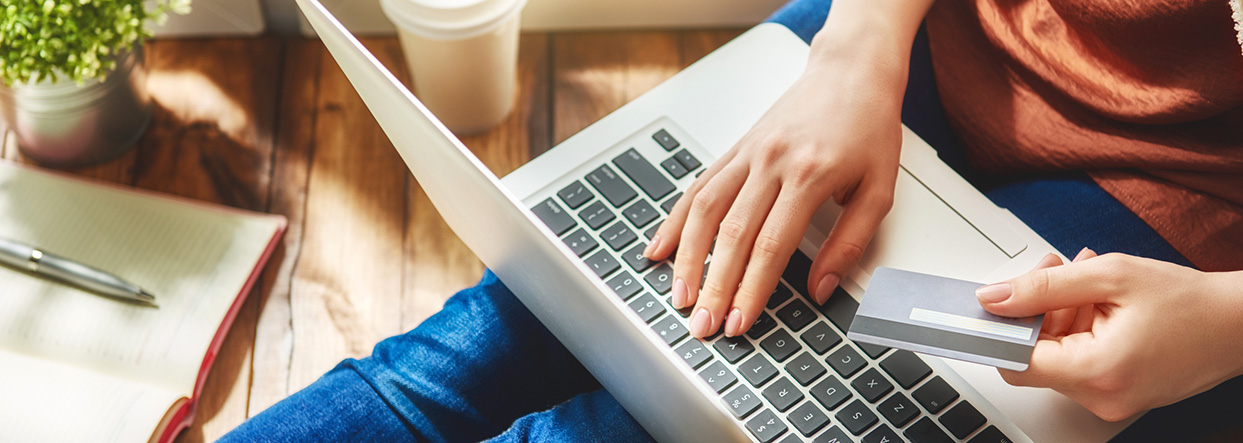
Recommendation systems with purchase data are introduced at specific points in the journey of shopping and at the end of the journey so that the customer feels fulfilled with their experience. For this, several players use decision tools. Called “automatic recommendation systems,” these tools analyze a customer’s purchase behavior and set the stage for digital ubiquity in retail. By employing complex algorithms, they help retailers identify products the customer may buy if they were to display it to the customer.
Let us look at how the recommendation engines really work towards creating and maintaining an enriching customer relationship.
#1. Wide Products at Disposal
Recommendation engines are data crunchers. They have complex algorithms that gather information on consumers behavior in digital retails by browsing history of customers. They highlight the best-suited and best-selling merchandise based on customer reviews and ratings. The right recommendation engine also aide retailers to send emails and keep the customers updated with the latest trends in the category of the purchased item. Thus, these engines create a long-term sustainable relationship with the customer.
#2. Concise Presentation of Products
Many retailers use recommendation engines for targeted marketing via email campaigns and website pages. Thus, they are capable of recommending several products from different categories. All these results are based on browsing history and have a higher likelihood of being bought when presented at the right time and in the right manner. These algorithms also browse through numerous merchandises listed in the retailer’s database, and present buyers a much narrow selection of items that they would likely purchase. Implementing artificial intelligence can save time and deliver a better user experience. This feature helps create a strong trust factor as customers know the retailer’s capabilities and how seriously they take this relationship.
#3. Customer Satisfaction
Recommendations engines make the journey to intelligent automation seamless for the retailers and much more enjoyable experience for the customers. In instances where the visitor left the site for some reason, the recommendation engines play a vital role in getting the attention of the customers back to the website. Features such as the availability of previous browsing data save time and energy of the visitor. It assists them to take the shopping activity further. The best recommendation engines make the whole process more engaging and increase the satisfaction rate of the visitors.
#4. Personalization
Machine learning and intelligent automation make recommendation engines act more like the customer’s friend or family. They are capable enough to give the right recommendations. As human nature, when shoppers feel indecisive about a purchase, they always look for a piece of advice from someone known to them. The trust factor comes from the fact that they know the buyer well and will give good recommendations. Product recommendation engines collect data to personalize offers and ensure that customers are happy with their purchase. Retailers can earn customers’ trust and loyalty by proving how well they understand them.
#5. Retain Happy Customers
AI recommendation engine in retail empowers retailers to present multiple relevant options to the customers that complement their requirements. It makes it easy for customers to avail loyalty points which in turn helps the retailer to retain customers and keep them engaged. Machine learning and data science technologies record, cleanse, normalize, and aggregate data which results in an extensive inventory of actionable recommendations for the customers.
AWS Neptune – The Graph Database Solution
Accelerating digital transformation in retail is Amazon Neptune. At the core of Amazon Neptune is a purpose-built, high-performance graph database engine. AWS Neptune is optimized for storing innumerable relationships and querying the graph with milliseconds latency. It makes it easy to build and run applications that work with highly connected datasets, as in the case of recommendation engines. Amazon Neptune stores relationships between information such as customer interests, friends, and purchase history in a graph and quickly queries it to make recommendations that are personalized and relevant. With AWS Neptune, retailers can use a highly available graph database to make product recommendations to a user. The recommendations are also based on merchandise purchased by others who have the same requirements and have similar purchase histories.
[caption id="attachment_13606" align="alignnone" width="600"] Happy pretty young Vietnamese shop assistant giving paper bag with order to female customer[/caption]
CONCLUSION
By going deeper into customers’ interests and preferences, product recommendation engines display more-relevant and predictive recommendations in today’s curt-throat retail digital transformation market. These tools are capable to access a broader range of merchandise to include niche items in shoppers’ recommendations.